This article delves into the accuracy of modern measurement tools used in the diagnosis and monitoring of Progressive Primary Aphasia (PPA) syndromes. The focus is on evaluating the effectiveness of diagnostic tools, standardized cognitive batteries, and wearable devices in identifying and tracking PPA.
Key Takeaways
- Accuracy of standardized cognitive batteries is crucial in correctly identifying and classifying patients with PPA syndromes.
- Development of brief and standardized assessment tools is essential for efficient PPA identification.
- Wearable devices equipped with sensor technology and machine learning algorithms show promise in disease detection and real-time monitoring.
- The accuracy of diagnostic tools for PPA diagnosis needs to be evaluated and standardized.
- Machine learning algorithms enhance the potential of wearable devices for early disease detection and monitoring.
Accuracy of Diagnostic Tools in Identifying PPA Syndromes
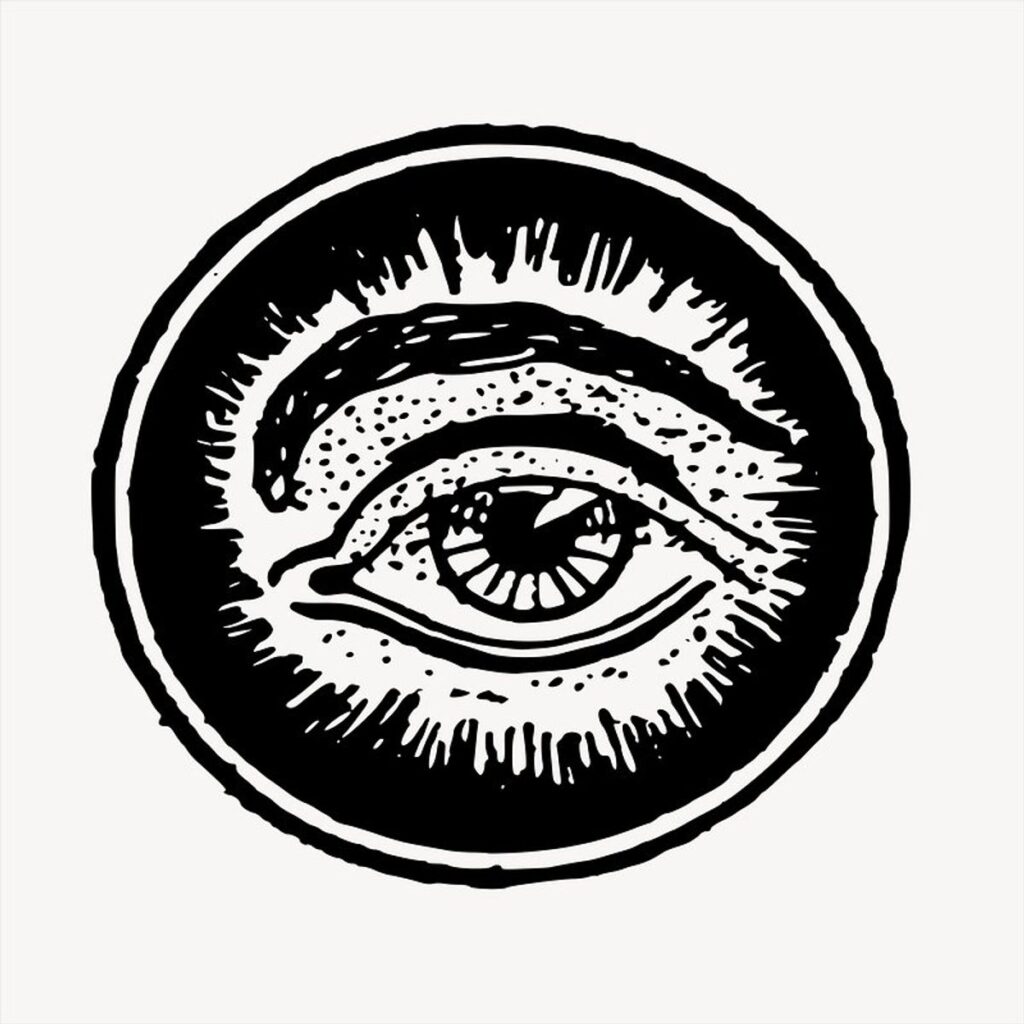
Research Question
The core of our investigation centers on the efficacy of modern PD (Pupillary Distance) measurement tools in the precise identification of Primary Progressive Aphasia (PPA) syndromes. This inquiry is crucial as it underpins the subsequent development and refinement of diagnostic methodologies.
To encapsulate the essence of our research question, we refer to the PIRD framework, which stands for Population, Index Test, Reference Test, and Diagnosis of Interest. The table below succinctly presents the research question structured according to this framework:
Our aim is to dissect the capabilities and limitations of current PD measurement technologies, such as Optigrid’s online SaaS platform, which promises to revolutionize optical measurements for eyeglasses businesses and optometrists, especially in the PPE eyewear industry.
The pursuit of this research question is not merely academic; it has profound implications for the quality of life of individuals affected by PPA, as accurate diagnosis is the gateway to targeted interventions and therapies.
Objectives
The primary aim of this study is to evaluate the precision and reliability of modern PD (Pupillary Distance) measurement tools in the context of PPA (Primary Progressive Aphasia) syndrome identification. To achieve this, the following objectives have been set:
- To assess the accuracy of current PD measurement technologies in clinical settings.
- To determine the effectiveness of these tools in enhancing the diagnostic process for PPA syndromes.
- To identify potential areas for improvement in PD measurement methodologies.
The overarching goal is to bridge the gap between technological advancements and their practical application in healthcare, particularly in the early detection and monitoring of PPA syndromes.
Recent innovations, such as the Optigrid system, have shown promise in revolutionizing optical measurements. This technology not only offers precise PD measurements but also incorporates user-friendly features that could significantly benefit the creation of PPE eyeglasses. By automating data capture and providing real-time feedback, tools like Optigrid could play a crucial role in enhancing both accuracy and customer service in the eyeglasses industry.
Methods and Analysis
The methodology for assessing the accuracy of modern PD measurement tools involves a multi-faceted approach. Formal analysis and data curation form the backbone of the research, ensuring that the data collected is both reliable and valid. The team, including experts like Rohan Khemlani and Shintato Nakayama, brings a wealth of experience in conceptualization and methodology to the project.
Statistical analysis plays a critical role in interpreting the results. Various methods such as Score Cut Point, Meaningful Change, and G-code Severity are employed to determine the significance of the findings. These methods help in linking the measures to real-world applications, providing a clear picture of the tools’ effectiveness.
The integration of advanced image analysis techniques allows for a more nuanced understanding of the data, leading to more accurate diagnoses and better patient outcomes.
The following table summarizes the key components of the methodology:
Development of Standardized Cognitive Batteries for PPA Identification
Current Tools
The landscape of tools for the identification of Primary Progressive Aphasia (PPA) has expanded significantly with the advent of advanced diagnostic technologies. Accurate PD and SH measurements are crucial for prescription eyewear and PPE, and by extension, for the precise assessment of cognitive functions affected by PPA. Tools such as Optigrid have revolutionized optical measurements, ensuring a perfect fit and optimal vision correction, which is essential for safety and compliance in both clinical and industrial settings.
The following table summarizes some of the key tools currently in use for PPA identification:
The integration of these tools into standardized cognitive batteries is imperative for enhancing the accuracy of PPA diagnosis.
In addition to these, there are various software frameworks and evaluation platforms that aid in the development and testing of new diagnostic approaches. The continuous improvement of these tools is vital for keeping pace with the evolving nature of PPA syndromes and the need for more nuanced detection methods.
Challenges
The journey towards perfecting cognitive batteries for PPA identification is fraught with challenges. Technological advancements have certainly pushed the boundaries of what’s possible, enhancing the accuracy of diagnostic tools. However, the integration of AI and other sophisticated technologies remains a complex task. Accurate measurement is crucial as it directly impacts the comfort and effectiveness of eyewear and vision correction for patients.
One of the primary challenges is the variability of PPA syndromes, which makes standardization difficult. Each case presents unique linguistic and cognitive impairments that require tailored assessment tools. Moreover, the interdisciplinary nature of PPA research necessitates collaboration across various fields, including neurology, speech therapy, and cognitive science.
The development of standardized cognitive batteries is essential for consistent and reliable PPA identification. This endeavor is not only a technical challenge but also a multidisciplinary one, requiring concerted efforts from experts in different domains.
Another significant hurdle is the lack of extensive datasets that are representative of the diverse population affected by PPA. This scarcity hampers the training of machine learning algorithms, which are pivotal for the future of automated and precise diagnostics.
Future Prospects
The evolution of PD measurement tools is poised to benefit significantly from advancements in technology. Optigrid technology, for instance, is set to revolutionize the precision of PD measurements. This innovation not only enhances accuracy but also simplifies the fitting process for PPE eyeglasses, accommodating a variety of designs with ease.
The integration of advanced technologies promises to deliver more reliable and user-friendly tools for practitioners and patients alike.
Looking ahead, the industry is moving towards a more personalized approach to PD measurement. The development of tools that can adapt to individual facial features and optical requirements is on the horizon. This personalization will likely lead to improved patient outcomes and satisfaction.
In summary, the future of PD measurement tools is bright, with new technologies paving the way for more accurate and adaptable solutions. The table below outlines potential areas of innovation and their expected impact on the field:
Utilization of Wearable Devices for Disease Detection and Monitoring
Sensor Technology
The advent of wearable devices has brought about a revolution in the field of health monitoring, with sensor technology at its core. These sensors are capable of tracking a wide range of biometric data, providing valuable insights into an individual’s health status.
Optical sensing technology, for example, includes Optical AFEs, Optical Mixed Signal Devices, and Optical Modules, which are integral to the functionality of wearable devices. Time of Flight (ToF) Sensors and Solutions offer another layer of data acquisition, enabling precise measurements of movement and spatial positioning.
Temperature sensing is another critical aspect, with devices utilizing Analog Temperature Sensors and Clinical-Grade Temperature Sensors to monitor body temperature fluctuations. This data is crucial for detecting fever or inflammation, which can be indicative of various health conditions.
In the context of Smart eyewear technology, augmented reality and health monitoring features are combined to enhance user experiences across various domains, including navigation and education, while also keeping track of biometric data.
The integration of advanced sensor technology in wearable devices has not only made continuous health monitoring feasible but also personalized, thanks to customization options and advancements like 3D printing.
Machine Learning Algorithms
The integration of machine learning algorithms with wearable devices has revolutionized the field of health monitoring. These algorithms are capable of processing complex data from various sensors to provide accurate and personalized health insights. For instance, regression and tree-based models like decision trees and random forests have been employed to predict daily step counts with a mean absolute error as low as 1908 steps.
Machine learning’s potential extends to highly accurate human activity recognition, with some models achieving up to 99.9% accuracy. This precision is critical for applications such as health monitoring and smart home management. Moreover, the use of machine learning in EMG signal classification and gait analysis has shown promising results in disease detection, including OCD and diabetes.
The sophistication of these algorithms allows for the development of systems that not only detect but also predict health events, enabling proactive interventions.
The table below summarizes the performance of various machine learning applications in wearable technology:
As the technology advances, we can expect even more sophisticated algorithms that will further enhance the accuracy and utility of wearable devices for health monitoring.
Real-Time Insights
The advent of wearable devices has ushered in a new era of health monitoring, where real-time insights are not just a possibility but a reality. These devices, equipped with advanced sensor technology, provide continuous data streams that enable proactive health management. For instance, smart contact lenses offer a glimpse into the future of personalized eye care, allowing for the monitoring of physiological parameters directly related to ocular health.
The integration of machine learning algorithms with wearable technology has been transformative. These algorithms analyze the vast amounts of data collected to detect patterns and anomalies that may indicate the onset of disease. This capability is particularly beneficial for patients with chronic conditions, as it facilitates the creation of personalized treatment plans tailored to their unique health profiles.
Remote monitoring solutions have revolutionized patient care by enhancing convenience and accessibility. Virtual optometrist tools, for example, allow for eye examinations and consultations to take place virtually, reducing the need for in-person visits. This not only saves time but also ensures that care is accessible to those in remote or underserved areas.
The potential of wearable devices to improve patient outcomes and streamline healthcare processes is immense. As technology continues to advance, we can expect these tools to become even more integrated into our daily lives, providing valuable health insights at our fingertips.
The advent of wearable devices has revolutionized the way we approach healthcare, offering unprecedented opportunities for disease detection and monitoring. These sophisticated gadgets are not just for tracking fitness; they are now powerful tools that can provide early warnings for a variety of health conditions. To learn more about how wearable technology can enhance your health and well-being, visit our website and discover the potential of these innovative devices. Don’t miss out on the cutting-edge developments in personal healthcare – stay informed and proactive with OPTIGRID.
Conclusion
In conclusion, the exploration of the accuracy of modern PD measurement tools has revealed significant advancements in the field. From the development of multimodal sensor gloves for Parkinson’s disease assessment to the utilization of electro-mechanical wearables for early disease detection, the potential of wearable devices in healthcare is evident. Additionally, the research on cognitive and language batteries for PPA diagnosis highlights the importance of standardized and brief assessment tools. Moving forward, further research and evaluation of these tools are essential to improve accuracy and efficiency in diagnosing and categorizing PPA syndromes.
Frequently Asked Questions
What is the primary research question of the present protocol?
The primary research question focuses on the accuracy of diagnostic tools in identifying and classifying patients with PPA syndromes.
What are the objectives of the study on cognitive batteries for PPA identification?
The objectives include comparing the accuracy of cognitive/aphasia batteries for diagnosing PPA variants according to current clinical criteria.
How are wearable devices utilized for disease detection and monitoring?
Wearable devices utilize sensor technology and machine learning algorithms to provide real-time insights for disease detection and monitoring.
What are the challenges in the development of standardized cognitive batteries for PPA identification?
Challenges include the need for specialized training, time-consuming application, and the lack of standardized assessment tools.
What are the future prospects of standardized cognitive batteries for PPA identification?
Future prospects include the development of brief and validated assessment tools for accurate classification of PPA syndromes.
How do machine learning algorithms contribute to the accuracy of wearable devices for disease detection?
Machine learning algorithms, such as support vector machine and k-nearest neighbors, enhance the accuracy of wearable devices by analyzing data from sensors and providing accurate disease detection results.
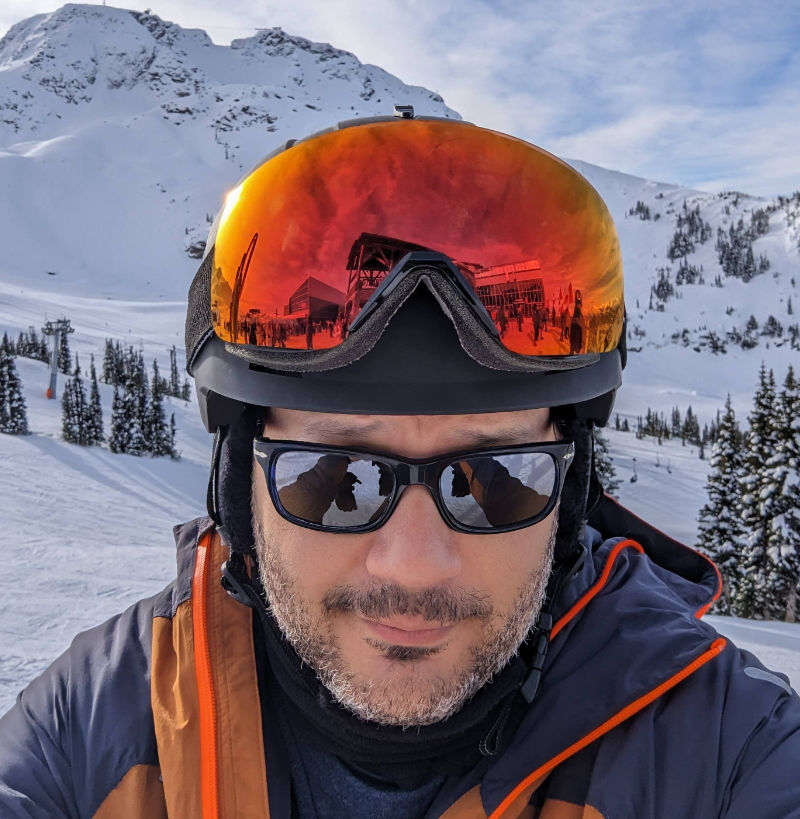
I am a seasoned software engineer with over two decades of experience and a deep-rooted background in the optical industry, thanks to a family business. Driven by a passion for developing impactful software solutions, I pride myself on being a dedicated problem solver who strives to transform challenges into opportunities for innovation.